Dental Restoration using a Multi-Resolution Deep Learning Approach
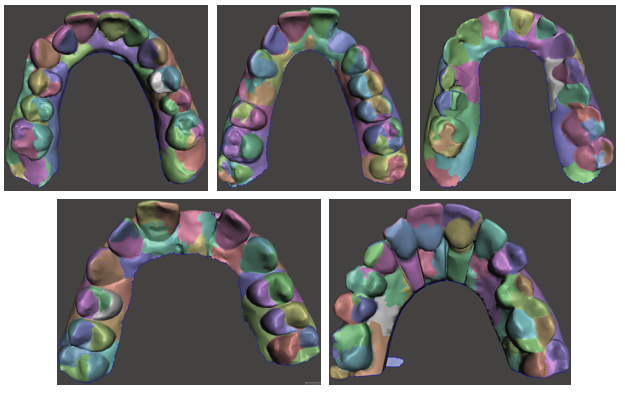
Published in SPIE. Digital Library, here’s an article published by our team explaining how our work in dental AI, through semi-supervised segmentation, improves the generalization of currently available deep learning segmentation model on 3D dental arches by introducing a new loss function to leverage unlabeled available data.
Dental offices tackle thousands of dental reconstructions every year. Complexity and abnormalities in dentition make segmentation of an optical scan a challenging manual task that takes 45 minutes on average. The present work improves the generalization of currently available deep learning segmentation model on 3D dental arches by introducing a new loss function to leverage unlabeled available data. The semi-supervised segmentation network is trained using a joint loss that combines a supervised loss of annotated input and a self-supervised loss of non-labeled input. Our results showed that combining self-supervised and supervised learning improved the segmentation score by 13 % compared with purely supervised learning for the same amount of labeled data. It is concluded that combining representations obtained from self-supervised learning with supervised learning improves the generalization of the 3D tooth segmentation model in the case of few available labeled data.
Ammar Alsheghri, Farnoosh Ghadiri, Ying Zhang, Olivier Lessard, Julia Keren, Farida Cheriet, François Guibault
You may read the full publication here.